动手搭建深度强化学习的自动股票量化交易系统
这个项目介绍并实现了深度强化学习算法,例如DDPG,TD3,SAC等算法,并支持推理部署。可以用于股票的量化交易,外汇量化交易,投资组合,金融产品推荐等场景。
基于深度强化学习的股票量化交易
⭐ ⭐ ⭐ 欢迎点个小小的Star支持!⭐ ⭐ ⭐
开源不易,希望大家多多支持~
- 更多实践案例(AI识虫,基于PaddleX实现森林火灾监测,眼疾识别,智能相册分类等)、深度学习资料,请参考:awesome-DeepLearning
- 更多学习资料请参阅飞桨深度学习平台
1.项目介绍
金融领域每天会产生大量的数据,这些数据的噪声性质很强,信息不全,很难利用起来进行分析。传统的随机控制理论和其他的分析方法在利用这些数据做决策的时候,这些方法会严重依赖模型的一些假设。但是强化学习能够利用这些每天产生的大量的金融数据,强化学习不需要对模型或者数据进行假设,并通过构建金融环境就能够学习到很复杂的金融决策策略,可以用于自动化股票辅助决策交易,投资组合,金融产品推荐等领域。
目前的股票交易策略有2种,第一种是价格预测,即使用机器学习的方法来预测未来的股价,交易就使用了一个预先定义好的交易策略,这个交易策略综合考虑了机器学习预测出来的价格,经纪人佣金,税费等等;第二种是自动化交易学习,即给定每天的股票的数据,直接学习交易策略使得获取的利润最大化。
1.1 项目内容
股票交易是一个经典的时序决策问题,其指的是在每个交易时间点通过分析历史图表,从而做出对应决策(如:买入、卖出、观望等),以达到长期的最大收益。因此,该问题可以被建模为一个强化学习问题。在此场景下,人即为智能体,股票市场为环境,人通过对股票做出决策,即与环境交互后,会获得股票当前的状态。
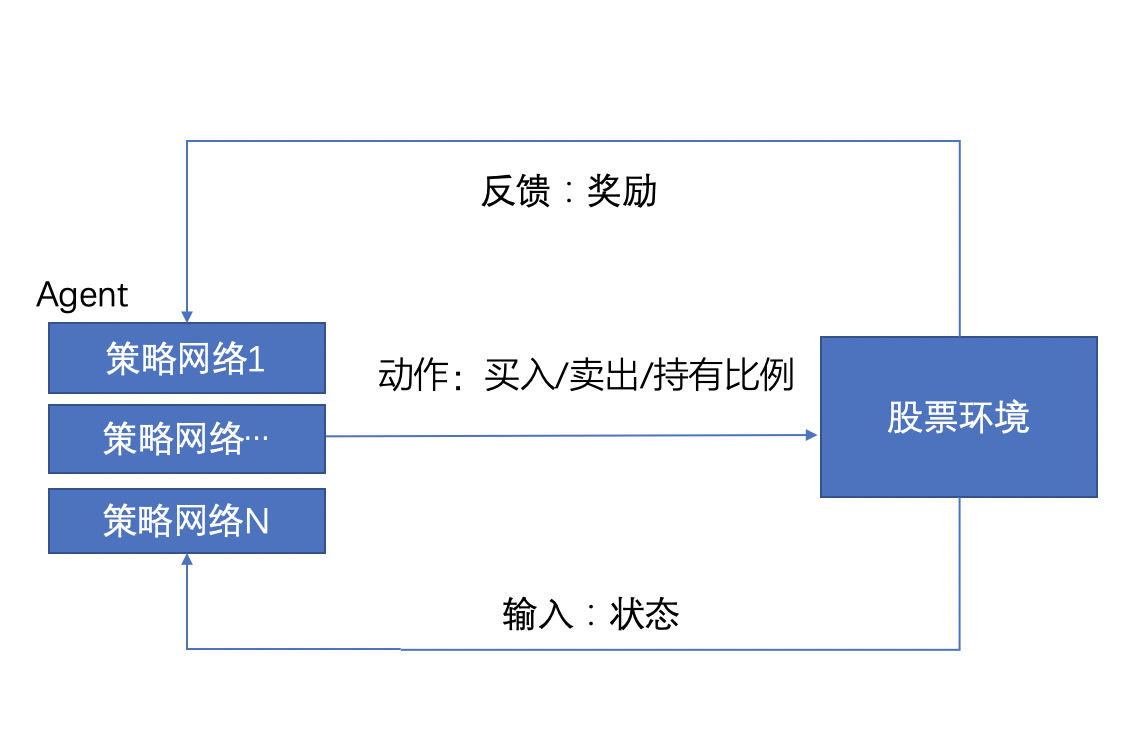
在此项目中,股票状态包含20个属性变量,包含所采用第三方股票数据包baostock
的一些股票属性和基于此计算得到的一些属性变量,分别为:
属性名 | 含义 |
---|---|
open | 当天开盘价格 |
high | 最高价格 |
low | 最低价格 |
close | 收盘价格 |
volume | 成交量 |
amount | 成交额 |
adjustflag | 赋权状态(1:后复权,2:前复权,3:不复权) |
tradestatus | 交易状态(1:正常交易,0:停牌) |
pctChg | 涨跌幅(百分比) |
peTTM | 滚动市盈率 |
pbMRQ | 市净率 |
psTTM | 滚动市销率 |
balance | 当前拥有的金钱 |
max_net_worth | 最大资产净值 |
net_worth | 当前资产净值 |
shares_held | 持有的手数 |
cost_basis | 即时买入价格 |
total_shares_sold | 总共抛出的手数 |
total_sales_value | 总共抛出的价值 |
NOTE
:上述属性值均会经过归一化处理,因此在此项目中,状态为一个长度为20的一维向量,其中每一个值的值域均为 [ 0 , 1 ] [0,1] [0,1]。
人根据当前的状态,依据现有的策略,执行相应的动作,在此项目中,可执行的动作为以下三种:
值区间 | 动作 |
---|---|
( 2 3 , 1 ) (\frac{2}{3},1) (32,1) | 卖出股票 |
( 1 3 , 2 3 ) (\frac{1}{3},\frac{2}{3}) (31,32) | 观望 |
( 0 , 1 3 ) (0,\frac{1}{3}) (0,31) | 买入股票 |
为了定量买入/卖出的股票数量,此项目加入了另一个值amount
,表示买入/卖出的股票的比例。因此,此场景下的动作空间为一个长度为2的一维向量,其中第一个值表示动作种类,值域为 [ 0 , 1 ] [0,1] [0,1];第二个值表示买入/卖出的股票的比例,值域为 [ 0 , 1 ] [0,1] [0,1]。
在该项目中,若触发以下三种情况任意一种,则一轮实验终止(我们称一个序幕(episode)为一轮实验):
- 最大资产净值大于等于最大金钱乘以最大预测的收益比,即:
m a x _ n e t _ w o r t h ≥ i n i t i a l _ a c c o u n t _ b a l a n c e × m a x _ p r e d i c t _ r a t e \mathbb{max\_net\_worth\ge{initial\_account\_balance\times{max\_predict\_rate}}} max_net_worth≥initial_account_balance×max_predict_rate
- 状态转移到数据集中的最后一天
- 当前的资产净值小于等于0,即:
n e t _ w o r t h ≤ 0 \mathbb{net\_worth\le0} net_worth≤0
该项目中的奖励信号reward设计基于相对初始收益比来度量,具体地:
- 计算出当前状态状态 s s s采取动作 a a a的资产净值
net_worth
,其由两部分构成:当前资产和当前持有股票的价值,即:
n e t _ w o r t h = b a l a n c e + n u m _ s h a r e s _ h e l d × c u r r e n t _ p r i c e \mathbb{net\_worth=balance+num\_shares\_held\times{current\_price}} net_worth=balance+num_shares_held×current_price
- 计算出相对收益比:
p r o f i t _ p e r c e n t = n e t _ w o r t h − i n i t i a l _ a c c o u n t _ b a l a n c e i n i t i a l _ a c c o u n t _ b a l a n c e \mathbb{profit\_percent=\frac{net\_worth-initial\_account\_balance}{initial\_account\_balance}} profit_percent=initial_account_balancenet_worth−initial_account_balance
- 奖励设计:若相对收益比大于等于0,则奖励信号取相对收益比与最大预测的收益比的商;反之,则此轮决策交互的奖励为-0.1。即有:
r e w a r d = { p r o f i t _ p e r c e n t m a x _ p r e d i c t _ r a t e , i f p r o f i t _ p e r c e n t > 0 − 0.1 , o t h e r s \mathbb{reward=} \begin{cases} \mathbb{\frac{profit\_percent}{max\_predict\_rate}},\quad{if\ }\mathbb{profit\_percent>0}\\ -0.1,\quad\quad\quad\quad\quad\quad\quad{others} \end{cases} reward={max_predict_rateprofit_percent,if profit_percent>0−0.1,others
2.安装说明
在进行项目之前,安装最新版本的parl。
!pip install parl==2.0.4 -i https://mirror.baidu.com/pypi/simple
Looking in indexes: https://mirror.baidu.com/pypi/simple
Requirement already satisfied: parl==2.0.4 in /opt/conda/envs/python35-paddle120-env/lib/python3.7/site-packages (2.0.4)
Requirement already satisfied: cloudpickle==1.6.0 in /opt/conda/envs/python35-paddle120-env/lib/python3.7/site-packages (from parl==2.0.4) (1.6.0)
Requirement already satisfied: scipy>=1.0.0 in /opt/conda/envs/python35-paddle120-env/lib/python3.7/site-packages (from parl==2.0.4) (1.6.3)
Requirement already satisfied: psutil>=5.6.2 in /opt/conda/envs/python35-paddle120-env/lib/python3.7/site-packages (from parl==2.0.4) (5.7.2)
Requirement already satisfied: grpcio>=1.27.2 in /opt/conda/envs/python35-paddle120-env/lib/python3.7/site-packages (from parl==2.0.4) (1.33.2)
Requirement already satisfied: protobuf>=3.14.0 in /opt/conda/envs/python35-paddle120-env/lib/python3.7/site-packages (from parl==2.0.4) (3.14.0)
Requirement already satisfied: click in /opt/conda/envs/python35-paddle120-env/lib/python3.7/site-packages (from parl==2.0.4) (7.1.2)
Requirement already satisfied: tb-nightly==1.15.0a20190801 in /opt/conda/envs/python35-paddle120-env/lib/python3.7/site-packages (from parl==2.0.4) (1.15.0a20190801)
Requirement already satisfied: pyzmq==18.1.1 in /opt/conda/envs/python35-paddle120-env/lib/python3.7/site-packages (from parl==2.0.4) (18.1.1)
Requirement already satisfied: termcolor>=1.1.0 in /opt/conda/envs/python35-paddle120-env/lib/python3.7/site-packages (from parl==2.0.4) (1.1.0)
Requirement already satisfied: flask>=1.0.4 in /opt/conda/envs/python35-paddle120-env/lib/python3.7/site-packages (from parl==2.0.4) (1.1.1)
Requirement already satisfied: requests in /opt/conda/envs/python35-paddle120-env/lib/python3.7/site-packages (from parl==2.0.4) (2.24.0)
Requirement already satisfied: flask-cors in /opt/conda/envs/python35-paddle120-env/lib/python3.7/site-packages (from parl==2.0.4) (3.0.8)
Requirement already satisfied: tensorboardX==1.8 in /opt/conda/envs/python35-paddle120-env/lib/python3.7/site-packages (from parl==2.0.4) (1.8)
Requirement already satisfied: setuptools>=41.0.0 in /opt/conda/envs/python35-paddle120-env/lib/python3.7/site-packages (from tb-nightly==1.15.0a20190801->parl==2.0.4) (56.2.0)
Requirement already satisfied: markdown>=2.6.8 in /opt/conda/envs/python35-paddle120-env/lib/python3.7/site-packages (from tb-nightly==1.15.0a20190801->parl==2.0.4) (3.1.1)
Requirement already satisfied: absl-py>=0.4 in /opt/conda/envs/python35-paddle120-env/lib/python3.7/site-packages (from tb-nightly==1.15.0a20190801->parl==2.0.4) (0.8.1)
Requirement already satisfied: six>=1.10.0 in /opt/conda/envs/python35-paddle120-env/lib/python3.7/site-packages (from tb-nightly==1.15.0a20190801->parl==2.0.4) (1.16.0)
Requirement already satisfied: numpy>=1.12.0 in /opt/conda/envs/python35-paddle120-env/lib/python3.7/site-packages (from tb-nightly==1.15.0a20190801->parl==2.0.4) (1.19.5)
Requirement already satisfied: wheel>=0.26 in /opt/conda/envs/python35-paddle120-env/lib/python3.7/site-packages (from tb-nightly==1.15.0a20190801->parl==2.0.4) (0.36.2)
Requirement already satisfied: werkzeug>=0.11.15 in /opt/conda/envs/python35-paddle120-env/lib/python3.7/site-packages (from tb-nightly==1.15.0a20190801->parl==2.0.4) (1.0.1)
Requirement already satisfied: Jinja2>=2.10.1 in /opt/conda/envs/python35-paddle120-env/lib/python3.7/site-packages (from flask>=1.0.4->parl==2.0.4) (2.11.3)
Requirement already satisfied: itsdangerous>=0.24 in /opt/conda/envs/python35-paddle120-env/lib/python3.7/site-packages (from flask>=1.0.4->parl==2.0.4) (1.1.0)
Requirement already satisfied: urllib3!=1.25.0,!=1.25.1,<1.26,>=1.21.1 in /opt/conda/envs/python35-paddle120-env/lib/python3.7/site-packages (from requests->parl==2.0.4) (1.25.6)
Requirement already satisfied: certifi>=2017.4.17 in /opt/conda/envs/python35-paddle120-env/lib/python3.7/site-packages (from requests->parl==2.0.4) (2019.9.11)
Requirement already satisfied: idna<3,>=2.5 in /opt/conda/envs/python35-paddle120-env/lib/python3.7/site-packages (from requests->parl==2.0.4) (2.8)
Requirement already satisfied: chardet<4,>=3.0.2 in /opt/conda/envs/python35-paddle120-env/lib/python3.7/site-packages (from requests->parl==2.0.4) (3.0.4)
Requirement already satisfied: MarkupSafe>=0.23 in /opt/conda/envs/python35-paddle120-env/lib/python3.7/site-packages (from Jinja2>=2.10.1->flask>=1.0.4->parl==2.0.4) (1.1.1)
[33mWARNING: You are using pip version 22.0.4; however, version 22.1.2 is available.
You should consider upgrading via the '/opt/conda/envs/python35-paddle120-env/bin/python -m pip install --upgrade pip' command.[0m[33m
[0m
!pip install -r requirements.txt
Looking in indexes: https://pypi.tuna.tsinghua.edu.cn/simple
Requirement already satisfied: paddle-serving-app>=0.7.0 in /opt/conda/envs/python35-paddle120-env/lib/python3.7/site-packages (from -r requirements.txt (line 1)) (0.9.0)
Requirement already satisfied: paddle-serving-client>=0.7.0 in /opt/conda/envs/python35-paddle120-env/lib/python3.7/site-packages (from -r requirements.txt (line 2)) (0.9.0)
Requirement already satisfied: paddle-serving-server-gpu>=0.7.0.post102 in /opt/conda/envs/python35-paddle120-env/lib/python3.7/site-packages (from -r requirements.txt (line 3)) (0.9.0.post1028)
Requirement already satisfied: pillow in /opt/conda/envs/python35-paddle120-env/lib/python3.7/site-packages (from paddle-serving-app>=0.7.0->-r requirements.txt (line 1)) (8.2.0)
Requirement already satisfied: sentencepiece<=0.1.96 in /opt/conda/envs/python35-paddle120-env/lib/python3.7/site-packages (from paddle-serving-app>=0.7.0->-r requirements.txt (line 1)) (0.1.96)
Requirement already satisfied: shapely in /opt/conda/envs/python35-paddle120-env/lib/python3.7/site-packages (from paddle-serving-app>=0.7.0->-r requirements.txt (line 1)) (1.8.2)
Requirement already satisfied: six>=1.10.0 in /opt/conda/envs/python35-paddle120-env/lib/python3.7/site-packages (from paddle-serving-app>=0.7.0->-r requirements.txt (line 1)) (1.16.0)
Requirement already satisfied: pyclipper in /opt/conda/envs/python35-paddle120-env/lib/python3.7/site-packages (from paddle-serving-app>=0.7.0->-r requirements.txt (line 1)) (1.3.0.post3)
Requirement already satisfied: opencv-python==3.4.17.61 in /opt/conda/envs/python35-paddle120-env/lib/python3.7/site-packages (from paddle-serving-app>=0.7.0->-r requirements.txt (line 1)) (3.4.17.61)
Requirement already satisfied: numpy>=1.14.5 in /opt/conda/envs/python35-paddle120-env/lib/python3.7/site-packages (from opencv-python==3.4.17.61->paddle-serving-app>=0.7.0->-r requirements.txt (line 1)) (1.19.5)
Requirement already satisfied: protobuf>=3.11.0 in /opt/conda/envs/python35-paddle120-env/lib/python3.7/site-packages (from paddle-serving-client>=0.7.0->-r requirements.txt (line 2)) (3.14.0)
Requirement already satisfied: requests in /opt/conda/envs/python35-paddle120-env/lib/python3.7/site-packages (from paddle-serving-client>=0.7.0->-r requirements.txt (line 2)) (2.24.0)
Requirement already satisfied: grpcio<=1.33.2 in /opt/conda/envs/python35-paddle120-env/lib/python3.7/site-packages (from paddle-serving-client>=0.7.0->-r requirements.txt (line 2)) (1.33.2)
Requirement already satisfied: grpcio-tools<=1.33.2 in /opt/conda/envs/python35-paddle120-env/lib/python3.7/site-packages (from paddle-serving-client>=0.7.0->-r requirements.txt (line 2)) (1.33.2)
Requirement already satisfied: click==7.1.2 in /opt/conda/envs/python35-paddle120-env/lib/python3.7/site-packages (from paddle-serving-server-gpu>=0.7.0.post102->-r requirements.txt (line 3)) (7.1.2)
Requirement already satisfied: MarkupSafe==1.1.1 in /opt/conda/envs/python35-paddle120-env/lib/python3.7/site-packages (from paddle-serving-server-gpu>=0.7.0.post102->-r requirements.txt (line 3)) (1.1.1)
Requirement already satisfied: Jinja2==2.11.3 in /opt/conda/envs/python35-paddle120-env/lib/python3.7/site-packages (from paddle-serving-server-gpu>=0.7.0.post102->-r requirements.txt (line 3)) (2.11.3)
Requirement already satisfied: flask<2.0.0,>=1.1.1 in /opt/conda/envs/python35-paddle120-env/lib/python3.7/site-packages (from paddle-serving-server-gpu>=0.7.0.post102->-r requirements.txt (line 3)) (1.1.1)
Requirement already satisfied: itsdangerous==1.1.0 in /opt/conda/envs/python35-paddle120-env/lib/python3.7/site-packages (from paddle-serving-server-gpu>=0.7.0.post102->-r requirements.txt (line 3)) (1.1.0)
Requirement already satisfied: Werkzeug==1.0.1 in /opt/conda/envs/python35-paddle120-env/lib/python3.7/site-packages (from paddle-serving-server-gpu>=0.7.0.post102->-r requirements.txt (line 3)) (1.0.1)
Requirement already satisfied: func-timeout in /opt/conda/envs/python35-paddle120-env/lib/python3.7/site-packages (from paddle-serving-server-gpu>=0.7.0.post102->-r requirements.txt (line 3)) (4.3.5)
Requirement already satisfied: pyyaml in /opt/conda/envs/python35-paddle120-env/lib/python3.7/site-packages (from paddle-serving-server-gpu>=0.7.0.post102->-r requirements.txt (line 3)) (5.1.2)
Requirement already satisfied: pytest in /opt/conda/envs/python35-paddle120-env/lib/python3.7/site-packages (from paddle-serving-server-gpu>=0.7.0.post102->-r requirements.txt (line 3)) (7.1.2)
Requirement already satisfied: py>=1.8.2 in /opt/conda/envs/python35-paddle120-env/lib/python3.7/site-packages (from pytest->paddle-serving-server-gpu>=0.7.0.post102->-r requirements.txt (line 3)) (1.11.0)
Requirement already satisfied: pluggy<2.0,>=0.12 in /opt/conda/envs/python35-paddle120-env/lib/python3.7/site-packages (from pytest->paddle-serving-server-gpu>=0.7.0.post102->-r requirements.txt (line 3)) (1.0.0)
Requirement already satisfied: tomli>=1.0.0 in /opt/conda/envs/python35-paddle120-env/lib/python3.7/site-packages (from pytest->paddle-serving-server-gpu>=0.7.0.post102->-r requirements.txt (line 3)) (2.0.1)
Requirement already satisfied: packaging in /opt/conda/envs/python35-paddle120-env/lib/python3.7/site-packages (from pytest->paddle-serving-server-gpu>=0.7.0.post102->-r requirements.txt (line 3)) (21.3)
Requirement already satisfied: attrs>=19.2.0 in /opt/conda/envs/python35-paddle120-env/lib/python3.7/site-packages (from pytest->paddle-serving-server-gpu>=0.7.0.post102->-r requirements.txt (line 3)) (21.4.0)
Requirement already satisfied: importlib-metadata>=0.12 in /opt/conda/envs/python35-paddle120-env/lib/python3.7/site-packages (from pytest->paddle-serving-server-gpu>=0.7.0.post102->-r requirements.txt (line 3)) (4.2.0)
Requirement already satisfied: iniconfig in /opt/conda/envs/python35-paddle120-env/lib/python3.7/site-packages (from pytest->paddle-serving-server-gpu>=0.7.0.post102->-r requirements.txt (line 3)) (1.1.1)
Requirement already satisfied: idna<3,>=2.5 in /opt/conda/envs/python35-paddle120-env/lib/python3.7/site-packages (from requests->paddle-serving-client>=0.7.0->-r requirements.txt (line 2)) (2.8)
Requirement already satisfied: chardet<4,>=3.0.2 in /opt/conda/envs/python35-paddle120-env/lib/python3.7/site-packages (from requests->paddle-serving-client>=0.7.0->-r requirements.txt (line 2)) (3.0.4)
Requirement already satisfied: urllib3!=1.25.0,!=1.25.1,<1.26,>=1.21.1 in /opt/conda/envs/python35-paddle120-env/lib/python3.7/site-packages (from requests->paddle-serving-client>=0.7.0->-r requirements.txt (line 2)) (1.25.6)
Requirement already satisfied: certifi>=2017.4.17 in /opt/conda/envs/python35-paddle120-env/lib/python3.7/site-packages (from requests->paddle-serving-client>=0.7.0->-r requirements.txt (line 2)) (2019.9.11)
Requirement already satisfied: typing-extensions>=3.6.4 in /opt/conda/envs/python35-paddle120-env/lib/python3.7/site-packages (from importlib-metadata>=0.12->pytest->paddle-serving-server-gpu>=0.7.0.post102->-r requirements.txt (line 3)) (4.2.0)
Requirement already satisfied: zipp>=0.5 in /opt/conda/envs/python35-paddle120-env/lib/python3.7/site-packages (from importlib-metadata>=0.12->pytest->paddle-serving-server-gpu>=0.7.0.post102->-r requirements.txt (line 3)) (3.8.0)
Requirement already satisfied: pyparsing!=3.0.5,>=2.0.2 in /opt/conda/envs/python35-paddle120-env/lib/python3.7/site-packages (from packaging->pytest->paddle-serving-server-gpu>=0.7.0.post102->-r requirements.txt (line 3)) (3.0.8)
[33mWARNING: You are using pip version 22.0.4; however, version 22.1.2 is available.
You should consider upgrading via the '/opt/conda/envs/python35-paddle120-env/bin/python -m pip install --upgrade pip' command.[0m[33m
[0m
如果安装不上,则需要clone源代码进行安装。切换到终端,然后执行下面的命令:
git clone https://github.com/PaddlePaddle/PARL.git
cd PARL
python setup.py install
在运行项目之前,我们首先导入一下相关的库包
import argparse
import os
import gym
import random
from gym import spaces
import numpy as np
import pandas as pd
from parl.utils import logger, tensorboard, ReplayMemory
import paddle
from parl.algorithms import SAC
[32m[06-29 11:43:51 MainThread @utils.py:73][0m paddlepaddle version: 2.3.0.
/opt/conda/envs/python35-paddle120-env/lib/python3.7/site-packages/parl/remote/communication.py:38: DeprecationWarning: 'pyarrow.default_serialization_context' is deprecated as of 2.0.0 and will be removed in a future version. Use pickle or the pyarrow IPC functionality instead.
context = pyarrow.default_serialization_context()
3.环境构建
继承gym.env
,并重写相应的接口即可,如__init__()
,reset()
,step()
等,代码的实现细节如下:
# 默认的一些数据,用于归一化属性值
MAX_ACCOUNT_BALANCE = 2147480 # 最大的账户财产
MAX_NUM_SHARES = 2147480 # 最大的手数
MAX_SHARE_PRICE = 5000 # 最大的单手价格
MAX_VOLUME = 1e9 # 最大的成交量
MAX_AMOUNT = 1e10 # 最大的成交额
MAX_OPEN_POSITIONS = 5 # 最大的持仓头寸
MAX_STEPS = 1000 # 最大的交互次数
MAX_DAY_CHANGE = 1 # 最大的日期改变
max_loss =-50000 # 最大的损失
max_predict_rate = 3 # 最大的预测率
INITIAL_ACCOUNT_BALANCE = 100000 # 初始的金钱
class StockTradingEnv(gym.Env):
"""A stock trading environment for OpenAI gym"""
metadata = {'render.modes': ['human']}
def __init__(self, df):
super(StockTradingEnv, self).__init__()
self.df = df
# self.reward_range = (0, MAX_ACCOUNT_BALANCE)
# 动作的可能情况:买入x%, 卖出x%, 观望
self.action_space = spaces.Box(
low=np.array([-1, -1]), high=np.array([1, 1]), dtype=np.float32)
# 环境状态的维度
self.observation_space = spaces.Box(
low=0, high=1, shape=(20,), dtype=np.float32)
self.current_step = 0
def seed(self, seed):
random.seed(seed)
np.random.seed(seed)
# 处理状态
def _next_observation(self):
# 有些股票数据缺失一些数据,处理一下
d10 = self.df.loc[self.current_step, 'peTTM'] / 100
d11 = self.df.loc[self.current_step, 'pbMRQ'] / 100
d12 = self.df.loc[self.current_step, 'psTTM'] / 100
if np.isnan(d10): # 某些数据是0.00000000e+00,如果是nan会报错
d10 = d11 = d12 = 0.00000000e+00
obs = np.array([
self.df.loc[self.current_step, 'open'] / MAX_SHARE_PRICE,
self.df.loc[self.current_step, 'high'] / MAX_SHARE_PRICE,
self.df.loc[self.current_step, 'low'] / MAX_SHARE_PRICE,
self.df.loc[self.current_step, 'close'] / MAX_SHARE_PRICE,
self.df.loc[self.current_step, 'volume'] / MAX_VOLUME,
self.df.loc[self.current_step, 'amount'] / MAX_AMOUNT,
self.df.loc[self.current_step, 'adjustflag'],
self.df.loc[self.current_step, 'tradestatus'] / 1,
self.df.loc[self.current_step, 'pctChg'] / 100,
d10,
d11,
d12,
self.df.loc[self.current_step, 'pcfNcfTTM'] / 100,
self.balance / MAX_ACCOUNT_BALANCE,
self.max_net_worth / MAX_ACCOUNT_BALANCE,
self.net_worth / MAX_ACCOUNT_BALANCE,
self.shares_held / MAX_NUM_SHARES,
self.cost_basis / MAX_SHARE_PRICE,
self.total_shares_sold / MAX_NUM_SHARES,
self.total_sales_value / (MAX_NUM_SHARES * MAX_SHARE_PRICE),
])
return obs
# 执行当前动作,并计算出当前的数据(如:资产等)
def _take_action(self, action):
# 随机设置当前的价格,其范围上界为当前时间点的价格
current_price = random.uniform(
self.df.loc[self.current_step, "low"], self.df.loc[self.current_step, "high"])
action_type = action[0]
amount = action[1]
if action_type < 1/3 and self.balance >= current_price: # 买入amount%
total_possible = int(self.balance / current_price)
shares_bought = int(total_possible * amount)
if shares_bought != 0.:
prev_cost = self.cost_basis * self.shares_held
additional_cost = shares_bought * current_price
self.balance -= additional_cost
self.cost_basis = (
prev_cost + additional_cost) / (self.shares_held + shares_bought)
self.shares_held += shares_bought
elif action_type > 2/3 and self.shares_held != 0: # 卖出amount%
shares_sold = int(self.shares_held * amount)
self.balance += shares_sold * current_price
self.shares_held -= shares_sold
self.total_shares_sold += shares_sold
self.total_sales_value += shares_sold * current_price
else:
pass
# 计算出执行动作后的资产净值
self.net_worth = self.balance + self.shares_held * current_price
if self.net_worth > self.max_net_worth:
self.max_net_worth = self.net_worth
if self.shares_held == 0:
self.cost_basis = 0
# 与环境交互
def step(self, action):
# 在环境内执行动作
self._take_action(action)
done = False
status = None
reward = 0
# 判断是否终止
self.current_step += 1
# delay_modifier = (self.current_step / MAX_STEPS)
# reward += delay_modifier
if self.net_worth >= INITIAL_ACCOUNT_BALANCE * max_predict_rate:
reward += max_predict_rate
status = f'[ENV] success at step {self.current_step}! Get {max_predict_rate} times worth.'
# self.current_step = 0
done = True
if self.current_step > len(self.df.loc[:, 'open'].values) - 1:
status = f'[ENV] Loop training. Max worth was {self.max_net_worth}, final worth is {self.net_worth}.'
# reward += (self.net_worth / INITIAL_ACCOUNT_BALANCE - max_predict_rate) / max_predict_rate
reward += self.net_worth / INITIAL_ACCOUNT_BALANCE
self.current_step = 0 # loop training
done = True
if self.net_worth <= 0 :
status = f'[ENV] Failure at step {self.current_step}. Loss all worth. Max worth was {self.max_net_worth}'
reward += -1
# self.current_step = 0
done = True
else:
# 计算相对收益比,并据此来计算奖励
profit = self.net_worth - INITIAL_ACCOUNT_BALANCE
# profit = self.net_worth - self.balance
profit_percent = profit / INITIAL_ACCOUNT_BALANCE
if profit_percent > 0:
reward += profit_percent / max_predict_rate
elif profit_percent == 0:
reward += -0.1
else:
reward += -0.1
obs = self._next_observation()
return obs, reward, done, {
'profit': self.net_worth,
'current_step': self.current_step,
'status': status
}
# 重置环境
def reset(self, new_df=None):
# 重置环境的变量为初始值
self.balance = INITIAL_ACCOUNT_BALANCE
self.net_worth = INITIAL_ACCOUNT_BALANCE
self.max_net_worth = INITIAL_ACCOUNT_BALANCE
self.shares_held = 0
self.cost_basis = 0
self.total_shares_sold = 0
self.total_sales_value = 0
# 传入环境数据集
if new_df:
self.df = new_df
# if self.current_step > len(self.df.loc[:, 'open'].values) - 1:
self.current_step = 0
return self._next_observation()
def get_obs(self, current_step):
d10 = self.df.loc[current_step, 'peTTM'] / 100
d11 = self.df.loc[current_step, 'pbMRQ'] / 100
d12 = self.df.loc[current_step, 'psTTM'] / 100
if np.isnan(d10): # 某些数据是0.00000000e+00,如果是nan会报错
d10 = d11 = d12 = 0.00000000e+00
obs = np.array([
self.df.loc[current_step, 'open'] / MAX_SHARE_PRICE,
self.df.loc[current_step, 'high'] / MAX_SHARE_PRICE,
self.df.loc[current_step, 'low'] / MAX_SHARE_PRICE,
self.df.loc[current_step, 'close'] / MAX_SHARE_PRICE,
self.df.loc[current_step, 'volume'] / MAX_VOLUME,
self.df.loc[current_step, 'amount'] / MAX_AMOUNT,
self.df.loc[current_step, 'adjustflag'],
self.df.loc[current_step, 'tradestatus'] / 1,
self.df.loc[current_step, 'pctChg'] / 100,
d10,
d11,
d12,
self.df.loc[current_step, 'pcfNcfTTM'] / 100,
self.balance / MAX_ACCOUNT_BALANCE,
self.max_net_worth / MAX_ACCOUNT_BALANCE,
self.net_worth / MAX_ACCOUNT_BALANCE,
self.shares_held / MAX_NUM_SHARES,
self.cost_basis / MAX_SHARE_PRICE,
self.total_shares_sold / MAX_NUM_SHARES,
self.total_sales_value / (MAX_NUM_SHARES * MAX_SHARE_PRICE),
])
return obs
# 显示环境至屏幕
def render(self, mode='human'):
# 打印环境信息
profit = self.net_worth - INITIAL_ACCOUNT_BALANCE
print('-'*30)
print(f'Step: {self.current_step}')
print(f'Balance: {self.balance}')
print(f'Shares held: {self.shares_held} (Total sold: {self.total_shares_sold})')
print(f'Avg cost for held shares: {self.cost_basis} (Total sales value: {self.total_sales_value})')
print(f'Net worth: {self.net_worth} (Max net worth: {self.max_net_worth})')
print(f'Profit: {profit}')
return profit
# 获得数据
df = pd.read_csv('./stock/train.csv')
# 根据数据集设置环境
env = StockTradingEnv(df)
# T得到环境的参数信息(如:状态和动作的维度)
state_dim = env.observation_space.shape[0]
action_dim = env.action_space.shape[0]
max_action = float(env.action_space.high[1])
max_step = len(df.loc[:, 'open'].values)
print(f'state: {state_dim}, action: {action_dim}, action max value: {max_action}, max step:{max_step}')
state: 20, action: 2, action max value: 1.0, max step:5125
# 获得数据
eval_df = pd.read_csv('./stock/test_v1.csv')
# 根据数据集设置环境
eval_env = StockTradingEnv(eval_df)
4.模型构建
模型构建部分主要实现智能提StockAgent,StockModel,StockAgent定义了模型的学习和参数更新方法,StockModel定义了模型的结构。
import parl
import paddle
import paddle.nn as nn
import paddle.nn.functional as F
class StockAgent(parl.Agent):
def __init__(self, algorithm):
super(StockAgent, self).__init__(algorithm)
self.alg.sync_target(decay=0)
def predict(self, obs):
obs = paddle.to_tensor(obs.reshape(1, -1), dtype='float32')
action = self.alg.predict(obs)
action_numpy = action.cpu().numpy()[0]
return action_numpy
def sample(self, obs):
obs = paddle.to_tensor(obs.reshape(1, -1), dtype='float32')
action, _ = self.alg.sample(obs)
action_numpy = action.cpu().numpy()[0]
return action_numpy
def learn(self, obs, action, reward, next_obs, terminal):
terminal = np.expand_dims(terminal, -1)
reward = np.expand_dims(reward, -1)
obs = paddle.to_tensor(obs, dtype='float32')
action = paddle.to_tensor(action, dtype='float32')
reward = paddle.to_tensor(reward, dtype='float32')
next_obs = paddle.to_tensor(next_obs, dtype='float32')
terminal = paddle.to_tensor(terminal, dtype='float32')
critic_loss, actor_loss = self.alg.learn(obs, action, reward, next_obs,
terminal)
return critic_loss, actor_loss
# clamp bounds for Std of action_log
# action网络输出的标准差的上界和下界
LOG_SIG_MAX = 1.0
LOG_SIG_MIN = -1e9
class StockModel(parl.Model):
def __init__(self, obs_dim, action_dim):
super(StockModel, self).__init__()
self.actor_model = Actor(obs_dim, action_dim)
self.critic_model = Critic(obs_dim, action_dim)
def policy(self, obs):
return self.actor_model(obs)
def value(self, obs, action):
return self.critic_model(obs, action)
def get_actor_params(self):
return self.actor_model.parameters()
def get_critic_params(self):
return self.critic_model.parameters()
class Actor(parl.Model):
def __init__(self, obs_dim, action_dim):
super(Actor, self).__init__()
self.l1 = nn.Linear(obs_dim, 256)
self.l2 = nn.Linear(256, 256)
self.mean_linear = nn.Linear(256, action_dim)
self.std_linear = nn.Linear(256, action_dim)
def forward(self, obs):
x = F.relu(self.l1(obs))
x = F.relu(self.l2(x))
act_mean = self.mean_linear(x)
act_std = self.std_linear(x)
act_log_std = paddle.clip(act_std, min=LOG_SIG_MIN, max=LOG_SIG_MAX)
return act_mean, act_log_std
class Critic(parl.Model):
def __init__(self, obs_dim, action_dim):
super(Critic, self).__init__()
# Q1 network
self.l1 = nn.Linear(obs_dim + action_dim, 256)
self.l2 = nn.Linear(256, 256)
self.l3 = nn.Linear(256, 1)
# Q2 network
self.l4 = nn.Linear(obs_dim + action_dim, 256)
self.l5 = nn.Linear(256, 256)
self.l6 = nn.Linear(256, 1)
def forward(self, obs, action):
x = paddle.concat([obs, action], 1)
# Q1
q1 = F.relu(self.l1(x))
q1 = F.relu(self.l2(q1))
q1 = self.l3(q1)
# Q2
q2 = F.relu(self.l4(x))
q2 = F.relu(self.l5(q2))
q2 = self.l6(q2)
return q1, q2
设置强化学习的超参数。
SEED = 0 # 随机种子
WARMUP_STEPS = 640
EVAL_EPISODES = 5 # 评估的轮数
MEMORY_SIZE = int(1e5) # 经验池的大小
BATCH_SIZE = 64 # 批次的大小
GAMMA = 0.995 # 折扣因子
TAU = 0.005 # 当前网络参数比例,用于更新目标网络
ACTOR_LR = 1e-4 # actor网络的参数
CRITIC_LR = 1e-4 # critic网络的参数
alpha = 0.2 # 熵正则化系数, SAC的参数
MAX_REWARD = -1e9 # 最大奖励
file_name = f'sac_Stock' # 模型保存的名字
定义SAC算法和Agent,其他的DDPG和TD3算法的定义类似。
# Initialize model, algorithm, agent, replay_memory
model = StockModel(state_dim, action_dim)
algorithm = SAC(
model,
gamma=GAMMA,
tau=TAU,
alpha=alpha,
actor_lr=ACTOR_LR,
critic_lr=CRITIC_LR)
agent = StockAgent(algorithm)
rpm = ReplayMemory(
max_size=MEMORY_SIZE, obs_dim=state_dim, act_dim=action_dim)
W0629 11:43:52.308462 7549 gpu_context.cc:278] Please NOTE: device: 0, GPU Compute Capability: 7.0, Driver API Version: 11.2, Runtime API Version: 10.1
W0629 11:43:52.312708 7549 gpu_context.cc:306] device: 0, cuDNN Version: 7.6.
5. 模型训练
模型的训练过程如下,我们在训练环境中进行训练,在测试环境中进行测试,取在测试环境中平均回报最大的参数进行保存。
# Runs policy for 5 episodes by default and returns average reward
# A fixed seed is used for the eval environment
eval_seed = [0, 53, 47, 99, 107, 1, 17, 57, 97, 179, 777]
@paddle.no_grad()
def run_evaluate_episodes(agent, env, eval_episodes):
avg_reward = 0.
for epi in range(eval_episodes):
obs = env.reset()
env.seed(eval_seed[epi])
done = False
while not done:
action = agent.predict(obs)
obs, reward, done, _ = env.step(action)
avg_reward += reward
avg_reward /= eval_episodes
print(f'Evaluator: the average reward is {avg_reward:.3f} over {eval_episodes} episodes.')
return avg_reward
# Run episode for training
def run_train_episode(agent, env, rpm,episode_num):
action_dim = env.action_space.shape[0]
obs = env.reset()
env.seed(SEED)
done = False
episode_reward = 0
episode_steps = 0
while not done:
episode_steps += 1
# Select action randomly or according to policy
if rpm.size() < WARMUP_STEPS:
action = np.random.uniform(-1, 1, size=action_dim)
else:
action = agent.sample(obs)
# action = agent.sample(obs)
action = (action+1.0)/2.0
next_obs, reward, done, info = env.step(action)
terminal = float(done)
# Store data in replay memory
rpm.append(obs, action, reward, next_obs, terminal)
obs = next_obs
episode_reward += reward
# Train agent after collecting sufficient data
if rpm.size() >= WARMUP_STEPS:
batch_obs, batch_action, batch_reward, batch_next_obs, batch_terminal = rpm.sample_batch(
BATCH_SIZE)
agent.learn(batch_obs, batch_action, batch_reward, batch_next_obs,
batch_terminal)
# print(f'Learner: Episode {episode_steps+1} done. The reward is {episode_reward:.3f}.')
# 打印信息
current_step = info['current_step']
print(f'Learner: Episode {episode_num} done. The reward is {episode_reward:.3f}.')
print(info['status'])
return episode_reward, episode_steps
总共训练train_total_steps数,每训练完一个episode,我们把模型放到测试集的环境进行评估,得到平均奖励,并保存平均奖励最大的模型。
def do_train(agent, env, rpm):
save_freq = 1
total_steps = 0
train_total_steps = 3e6
episode_num = 0
best_award = -1e9
while total_steps < train_total_steps:
episode_num +=1
# Train episode
episode_reward, episode_steps = run_train_episode(agent, env, rpm,episode_num)
total_steps += episode_steps
if(episode_num%save_freq==0):
avg_reward = run_evaluate_episodes(agent, eval_env, EVAL_EPISODES)
if(best_award<avg_reward):
best_award = avg_reward
print(f'Saving best model!')
agent.save(f"./models/{file_name}.ckpt")
do_train(agent, env, rpm)
运行的时间比较长,需要耐心的等待。起始资金设置的是10万,大家可以从日志中看出收益,总体来说收益都是正向的,即大于10万。
6. 交易测试
交易测试环节加载最好的模型,并设置最大执行的数max_action_step,可以查看平均收益。
def run_test_episodes(agent, env, eval_episodes,max_action_step = 200):
avg_reward = 0.
avg_worth = 0.
for _ in range(eval_episodes):
obs = env.reset()
env.seed(0)
done = False
t = 0
while not done:
action = agent.predict(obs)
obs, reward, done, info = env.step(action)
avg_reward += reward
t+=1
if(t==max_action_step):
# eval_env.render()
print('over')
break
avg_worth += info['profit']
avg_reward /= eval_episodes
avg_worth /= eval_episodes
print(f'Evaluator: The average reward is {avg_reward:.3f} over {eval_episodes} episodes.')
print(f'Evaluator: The average worth is {avg_worth:.3f} over {eval_episodes} episodes.')
return avg_reward
# 获得数据
df = pd.read_csv('./stock/test_v1.csv')
# 根据数据集设置环境
env = StockTradingEnv(df)
agent.restore('models/sac_Stock_base.ckpt')
# 设置的最大执行的天数,每一个step表示一天
max_action_step = 400
avg_reward = run_test_episodes(agent, env, EVAL_EPISODES,max_action_step)
Evaluator: The average reward is 75.724 over 5 episodes.
Evaluator: The average worth is 210542.472 over 5 episodes.
7.线上部署
线上部署首先需要把强化学习模型导出,然后弄成serving的形式,然后集成到量化交易系统,就可以尝试使用看收益啦。
7.1 转换成静态图
利用parl库的save_inference_model接口把模型的actor网络部分转换成静态图。
save_inference_path = './output/inference_model'
input_shapes = [[None, env.observation_space.shape[0]]]
input_dtypes = ['float32']
agent.save_inference_model(save_inference_path, input_shapes, input_dtypes,
model.actor_model)
7.2 静态图预测
转换成静态图以后,接下来可以加载静态图模型进行简单的测试,给模型传入某一天的state的数据,然后模型预测出执行的动作。
from paddle import inference
class Predictor(object):
def __init__(self,
model_dir,
device="gpu",
batch_size=32,
use_tensorrt=False,
precision="fp32",
cpu_threads=10,
enable_mkldnn=False):
self.batch_size = batch_size
model_file = model_dir + "/inference_model.pdmodel"
params_file = model_dir + "/inference_model.pdiparams"
if not os.path.exists(model_file):
raise ValueError("not find model file path {}".format(model_file))
if not os.path.exists(params_file):
raise ValueError("not find params file path {}".format(params_file))
config = paddle.inference.Config(model_file, params_file)
if device == "gpu":
# set GPU configs accordingly
# such as intialize the gpu memory, enable tensorrt
config.enable_use_gpu(100, 0)
precision_map = {
"fp16": inference.PrecisionType.Half,
"fp32": inference.PrecisionType.Float32,
"int8": inference.PrecisionType.Int8
}
precision_mode = precision_map[precision]
if use_tensorrt:
config.enable_tensorrt_engine(max_batch_size=batch_size,
min_subgraph_size=30,
precision_mode=precision_mode)
elif device == "cpu":
# set CPU configs accordingly,
# such as enable_mkldnn, set_cpu_math_library_num_threads
config.disable_gpu()
if args.enable_mkldnn:
# cache 10 different shapes for mkldnn to avoid memory leak
config.set_mkldnn_cache_capacity(10)
config.enable_mkldnn()
config.set_cpu_math_library_num_threads(args.cpu_threads)
elif device == "xpu":
# set XPU configs accordingly
config.enable_xpu(100)
config.switch_use_feed_fetch_ops(False)
self.predictor = paddle.inference.create_predictor(config)
self.input_handles = [
self.predictor.get_input_handle(name)
for name in self.predictor.get_input_names()
]
# self.output_handle = self.predictor.get_output_handle(
# self.predictor.get_output_names()[0])
self.output_handle = [self.predictor.get_output_handle(name)
for name in self.predictor.get_output_names()]
# 重置环境的变量为初始值
self.balance = INITIAL_ACCOUNT_BALANCE
self.net_worth = INITIAL_ACCOUNT_BALANCE
self.max_net_worth = INITIAL_ACCOUNT_BALANCE
self.shares_held = 0
self.cost_basis = 0
self.total_shares_sold = 0
self.total_sales_value = 0
def predict(self, df):
"""
Predicts the data labels.
Args:
data (obj:`List(str)`): The batch data whose each element is a raw text.
tokenizer(obj:`PretrainedTokenizer`): This tokenizer inherits from :class:`~paddlenlp.transformers.PretrainedTokenizer`
which contains most of the methods. Users should refer to the superclass for more information regarding methods.
Returns:
results(obj:`dict`): All the predictions probs.
"""
obs = self.get_obs(df,0)
print(obs)
self.input_handles[0].copy_from_cpu(obs.reshape(1, -1).astype('float32'))
self.predictor.run()
action = self.output_handle[0].copy_to_cpu()
std = self.output_handle[1].copy_to_cpu()
return [action,std]
def get_obs(self, df, current_step):
self.df = df
d10 = self.df.loc[current_step, 'peTTM'] / 100
d11 = self.df.loc[current_step, 'pbMRQ'] / 100
d12 = self.df.loc[current_step, 'psTTM'] / 100
if np.isnan(d10): # 某些数据是0.00000000e+00,如果是nan会报错
d10 = d11 = d12 = 0.00000000e+00
obs = np.array([
self.df.loc[current_step, 'open'] / MAX_SHARE_PRICE,
self.df.loc[current_step, 'high'] / MAX_SHARE_PRICE,
self.df.loc[current_step, 'low'] / MAX_SHARE_PRICE,
self.df.loc[current_step, 'close'] / MAX_SHARE_PRICE,
self.df.loc[current_step, 'volume'] / MAX_VOLUME,
self.df.loc[current_step, 'amount'] / MAX_AMOUNT,
self.df.loc[current_step, 'adjustflag'],
self.df.loc[current_step, 'tradestatus'] / 1,
self.df.loc[current_step, 'pctChg'] / 100,
d10,
d11,
d12,
self.df.loc[current_step, 'pcfNcfTTM'] / 100,
self.balance / MAX_ACCOUNT_BALANCE,
self.max_net_worth / MAX_ACCOUNT_BALANCE,
self.net_worth / MAX_ACCOUNT_BALANCE,
self.shares_held / MAX_NUM_SHARES,
self.cost_basis / MAX_SHARE_PRICE,
self.total_shares_sold / MAX_NUM_SHARES,
self.total_sales_value / (MAX_NUM_SHARES * MAX_SHARE_PRICE),
])
return obs
model_dir = 'output'
device = 'gpu'
predictor = Predictor(model_dir, device)
df = pd.read_csv('./stock/test_v1.csv')
act_out, act_std = predictor.predict(df)
# print(result)
action = (act_out[0]+1.0)/2.0
print(act_out)
print(action)
[1.92800000e-03 1.94600000e-03 1.91000000e-03 1.93800000e-03
6.29069390e-02 6.06364959e-02 3.00000000e+00 1.00000000e+00
1.03300000e-03 5.14297900e-02 5.57414000e-03 1.47343800e-02
3.46801300e-02 4.65662078e-02 4.65662078e-02 4.65662078e-02
0.00000000e+00 0.00000000e+00 0.00000000e+00 0.00000000e+00]
[[-0.16079684 -0.09829579]]
[0.4196016 0.4508521]
[1m[35m--- Running analysis [ir_graph_build_pass][0m
[1m[35m--- Running analysis [ir_graph_clean_pass][0m
[1m[35m--- Running analysis [ir_analysis_pass][0m
[32m--- Running IR pass [is_test_pass][0m
[32m--- Running IR pass [simplify_with_basic_ops_pass][0m
[32m--- Running IR pass [conv_bn_fuse_pass][0m
[32m--- Running IR pass [conv_eltwiseadd_bn_fuse_pass][0m
[32m--- Running IR pass [embedding_eltwise_layernorm_fuse_pass][0m
[32m--- Running IR pass [multihead_matmul_fuse_pass_v2][0m
[32m--- Running IR pass [gpu_cpu_squeeze2_matmul_fuse_pass][0m
[32m--- Running IR pass [gpu_cpu_reshape2_matmul_fuse_pass][0m
[32m--- Running IR pass [gpu_cpu_flatten2_matmul_fuse_pass][0m
[32m--- Running IR pass [gpu_cpu_map_matmul_v2_to_mul_pass][0m
I0629 11:50:33.165313 7549 fuse_pass_base.cc:57] --- detected 4 subgraphs
[32m--- Running IR pass [gpu_cpu_map_matmul_v2_to_matmul_pass][0m
[32m--- Running IR pass [gpu_cpu_map_matmul_to_mul_pass][0m
[32m--- Running IR pass [fc_fuse_pass][0m
I0629 11:50:33.166007 7549 fuse_pass_base.cc:57] --- detected 4 subgraphs
[32m--- Running IR pass [fc_elementwise_layernorm_fuse_pass][0m
[32m--- Running IR pass [conv_elementwise_add_act_fuse_pass][0m
[32m--- Running IR pass [conv_elementwise_add2_act_fuse_pass][0m
[32m--- Running IR pass [conv_elementwise_add_fuse_pass][0m
[32m--- Running IR pass [transpose_flatten_concat_fuse_pass][0m
[32m--- Running IR pass [runtime_context_cache_pass][0m
[1m[35m--- Running analysis [ir_params_sync_among_devices_pass][0m
I0629 11:50:33.167120 7549 ir_params_sync_among_devices_pass.cc:100] Sync params from CPU to GPU
[1m[35m--- Running analysis [adjust_cudnn_workspace_size_pass][0m
[1m[35m--- Running analysis [inference_op_replace_pass][0m
[1m[35m--- Running analysis [ir_graph_to_program_pass][0m
I0629 11:50:33.170668 7549 analysis_predictor.cc:1007] ======= optimize end =======
I0629 11:50:33.170722 7549 naive_executor.cc:102] --- skip [feed], feed -> obs
I0629 11:50:33.170990 7549 naive_executor.cc:102] --- skip [linear_12.tmp_1], fetch -> fetch
I0629 11:50:33.170997 7549 naive_executor.cc:102] --- skip [clip_0.tmp_0], fetch -> fetch
7.3 Paddle Serving 部署
import paddle_serving_client.io as serving_io
dirname="output"
# 模型的路径
model_filename="inference_model.pdmodel"
# 参数的路径
params_filename="inference_model.pdiparams"
# server的保存地址
server_path="serving_server"
# client的保存地址
client_path="serving_client"
# 指定输出的别名
feed_alias_names=None
# 制定输入的别名
fetch_alias_names='mean_output,std_output'
# 设置为True会显示日志
show_proto=None
serving_io.inference_model_to_serving(
dirname=dirname,
serving_server=server_path,
serving_client=client_path,
model_filename=model_filename,
params_filename=params_filename,
show_proto=show_proto,
feed_alias_names=feed_alias_names,
fetch_alias_names=fetch_alias_names)
/opt/conda/envs/python35-paddle120-env/lib/python3.7/site-packages/paddle_serving_client/httpclient.py:22: DeprecationWarning: Using or importing the ABCs from 'collections' instead of from 'collections.abc' is deprecated, and in 3.8 it will stop working
from collections import Iterable
(dict_keys(['obs']), dict_keys(['linear_12.tmp_1', 'clip_0.tmp_0']))
搭建结束以后,就可以启动server部署服务,使用client端访问server端就行了。具体细节参考代码:https://github.com/PaddlePaddle/Serving/tree/v0.9.0/examples/Pipeline/simple_web_service
7.4 量化交易系统搭建
量化交易系统搭建请参考链接:https://github.com/vnpy/vnpy ,
VeighNa是一套基于Python的开源量化交易系统开发框架,在开源社区持续不断的贡献下一步步成长为多功能量化交易平台,自发布以来已经积累了众多来自金融机构或相关领域的用户,包括私募基金、证券公司、期货公司等。具有以下的特点:
1.丰富接口:支持大量高性能交易Gateway接口,包括:期货、期权、股票、期货期权、黄金T+d、银行间固收、外盘市场等
2.开箱即用:内置诸多成熟的量化交易策略App模块,用户可以自由选择通过GUI图形界面模式管理,或者使用CLI脚本命令行模式运行
3.自由拓展:结合事件驱动引擎的核心架构以及Python的胶水语言特性,用户可以根据自己的需求快速对接新的交易接口或者开发上层策略应用
4.开源平台:遵循开放灵活的MIT开源协议,可以在Gitee上获取所有项目源代码,自由使用于自己的开源项目或者商业项目,且永久免费
【注意】本项目从头到尾讲解了SAC算法应用,很容易实现多种强化学习的算法,然后可以综合决策,提升策略的鲁棒性
8.参考文献
[1].【协同育人项目】【实践】基于DDPG算法的股票量化交易. https://aistudio.baidu.com/aistudio/projectdetail/2221634
此文仅为搬运,原作链接:https://aistudio.baidu.com/aistudio/projectdetail/4275734?channelType=0&channel=0
更多推荐
所有评论(0)